Observability 101 Course
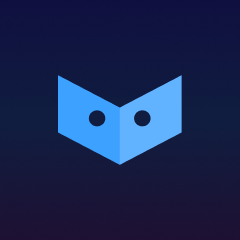
Observo.ai Team
Welcome to Observability 101, Observo AI's comprehensive guide to unveiling the mysteries of observability. Have you ever wanted to know something specific about observability but were afraid to ask? Fear not, this series is designed to be your go-to resource, answering all of the questions you might have. We add new chapters frequently, so check back to learn something new.
Syllabus
Chapter 1
What is Observability?
Explore observability, the capability to comprehend system behavior through the collection and analysis of telemetry data, essential for improving performance monitoring, troubleshooting, security monitoring, and capacity planning across various domains.
Chapter 2
Unlocking Enterprise Success: 10 Benefits of Observability
The ten key benefits of observability, emphasizing its role in enhancing system reliability, performance, and security, while also facilitating faster issue resolution, improved resource utilization, and cost optimization in complex IT environments.
Chapter 3
Exploring the Top 5 Use Cases for Observability: Navigating the Modern Tech Landscape
The top five observability use cases, including its pivotal role in optimizing system performance, troubleshooting issues, enhancing security monitoring, facilitating capacity planning, and ensuring the health of applications and infrastructure.
Chapter 4
What is an Observability Pipeline?
An observability pipeline is a framework that facilitates the collection, processing, and analysis of telemetry data, leveraging AI and automation to enhance observability and streamline operations across complex IT environments.
Chapter 5
Understanding Logs, Metrics, Events, and Traces: The Pillars of Observability
Explore the distinction between logs, metrics, events, and traces, their roles in providing comprehensive insights into system behavior, crucial for effective observability and troubleshooting in IT environments.
Chapter 6
What is Telemetry?
Telemetry is the data generated by various components within IT systems, encompassing metrics, logs, events, and traces, crucial for gaining insights into system behavior and ensuring effective observability and security.
Chapter 7
The Ten Key Principles of Telemetry and Observability for SaaS and Cloud Infrastructure
The ten principles of observability tailored for SaaS and cloud environments, emphasizing the importance of real-time insights, automation, scalability, and comprehensive data collection to ensure effective monitoring and troubleshooting capabilities in dynamic IT landscapes.
Chapter 8
Navigating the Telemetry Data and Observability Maze in Enterprises
Learn about the intricacies of managing telemetry data and observability, providing strategies for efficiently navigating the complexities inherent in diverse data sources to optimize system comprehension and operational effectiveness within IT environments.
Chapter 9
What is Open Telemetry?
OpenTelemetry (OTel) is a unified observability framework aimed at standardizing telemetry data collection across cloud-native applications, enhancing interoperability and simplifying instrumentation for monitoring and troubleshooting purposes in modern IT environments.
Chapter 10
OpenTelemetry: Elevating Observability and Log Management
OpenTelemetry (OTel) contributes to observability and log management by providing standardized instrumentation and data collection practices, facilitating comprehensive system monitoring and troubleshooting across diverse cloud-native environments.
Chapter 11
Demystifying Application Performance Monitoring (APM)
Explore Application Performance Monitoring (APM) and its role in tracking and optimizing the performance of software applications, highlighting its importance in ensuring optimal user experiences and operational efficiency within IT environments.
Chapter 12
Observability vs. Monitoring: Unraveling the Path to Insightful Operations
Learn about the distinction between observability and monitoring, highlighting observability's broader scope in understanding system behavior through telemetry data analysis, compared to monitoring's focus on specific metrics and thresholds for system health assessment within IT environments.
Chapter 13
Difference between APM and Log Management
Learn the differences between Application Performance Monitoring (APM) and log management, emphasizing APM's focus on monitoring application performance metrics and user experience, while log management primarily deals with storing and analyzing log data for troubleshooting, and auditing purposes within IT infrastructures.
Chapter 14
Why Log Management is Crucial for Business Success
Uncover the criticality of log management for business success, elucidating how effective log management enables organizations to gain valuable insights, ensure regulatory compliance, troubleshoot issues, and enhance security in dynamic IT environments.
Chapter 15
Understanding the Fundamentals of Logging in IT Systems
Fundamentals of logging in IT: its significance in recording system events, troubleshooting issues, monitoring performance, and maintaining security, essential for effective system management and operational resilience.
Chapter 16
What is a SIEM?
A SIEM (Security Information and Event Management) system is a comprehensive security solution that collects, analyzes, and correlates security event logs from various sources to detect and respond to security threats effectively within IT environments.
Chapter 17
SIEM vs. Log Management: Unraveling the World of Telemetry, Observability, and AI
SIEM (Security Information and Event Management) vs. monitoring, highlighting SIEM's focus on security event analysis and response, whereas monitoring primarily tracks system performance metrics, providing insights for operational optimization within IT infrastructures.
Chapter 18
Integrating Log Management with SIEM for Enhanced Security
The differences between SIEM (Security Information and Event Management) and monitoring, emphasizing SIEM's role in security event analysis and response, contrasting with monitoring's focus on system performance metrics for operational insights within IT environments.
Chapter 19
The Evolution of Observability: From Log Management to AI-Driven Analytics
The evolution of observability from traditional log management to AI-driven analytics, how advancements in technology have enabled more efficient and insightful approaches to understanding system behavior within IT infrastructures.
Chapter 20
Leveraging AI in Modern SIEM Architecture for Proactive Security
Leverage AI in modern SIEM (Security Information and Event Management) systems to enhance proactive security measures. AI-driven analytics can improve threat detection and response capabilities within IT environments.
Chapter 21
What are the differences Between SIEM, SoC, and SOAR
Distinguish between SIEM (Security Information and Event Management), SOC (Security Operations Center), and SOAR (Security Orchestration, Automation, and Response), highlighting their respective roles in security monitoring, incident management, and automated response within IT security operations.
Chapter 22
The Vital Importance of Data in Cybersecurity and how to get it right
Underscore the significance of data in cybersecurity, and how comprehensive data collection, analysis, and interpretation are essential for detecting and mitigating security threats effectively within IT environments.
Chapter 23
The critical role of Logs in modern Cybersecurity
The critical role of logs in modern cybersecurity, illustrating how thorough log management facilitates threat detection, incident response, forensic analysis, and compliance adherence within IT infrastructures.
Chapter 24
What are Security event logs?
Security event logs are records of security-related incidents and activities within IT systems, crucial for monitoring, analyzing, and responding to security threats effectively in cybersecurity operations.
Chapter 25
The Crucial Role of VPC Flow Logs in Enhancing Security and Ensuring Compliance
The role of VPC (Virtual Private Cloud) flow logs in enhancing security and compliance within cloud environments, illustrating how they provide valuable insights into network traffic, aiding in threat detection, incident response, and regulatory compliance efforts.
Chapter 26
The Critical Role of Firewall and Security Event Log Data in Cybersecurity and Compliance
The importance of firewall logs in maintaining cybersecurity and regulatory compliance, how they offer insights into network traffic, facilitate threat detection, incident response, and adherence to compliance standards within IT environments.
Chapter 27
Understanding OCSF - The Open Cybersecurity Schema Framework
OCSF (Open Cybersecurity Schema Framework) is an initiative aimed at standardizing cybersecurity telemetry data across various tools and platforms, allowing for easier data integration and analysis within observability pipelines. It provides a common language for security events, reducing the complexity of correlating data from multiple sources, and enhancing the effectiveness of security operations by enabling more accurate threat detection and response. Understanding OCSF is crucial for organizations looking to streamline their observability processes and improve overall cybersecurity posture.
Chapter 28
The Essential Role of Data Privacy and Data Confidentiality in Log Management and Observability
The intersection of data privacy, confidentiality, and observability, the importance of implementing privacy safeguards and encryption measures to protect sensitive data while maintaining observability within IT systems and infrastructure.
Chapter 29
What is Sensitive Data Discovery and Why is it Important in Observability and Logging?
Sensitive data discovery Is the process of identifying and categorizing sensitive information within IT systems, crucial for ensuring compliance with data protection regulations and implementing appropriate security measures to safeguard sensitive data from unauthorized access or disclosure.
Chapter 30
Unlocking Security Engineering Standards
The importance of unlocking security engineering standards, their role in establishing best practices, ensuring consistency, and promoting collaboration among security teams to enhance overall cybersecurity within organizations.
Chapter 31
Log Retention Requirements for Regulatory Compliance
Log retention requirements for regulatory compliance, adhering to specific data retention periods and storage practices to meet regulatory standards and facilitate effective auditing and incident response within organizations.
Chapter 32
Unlocking the Power of Observability Data Lakes
Observability data lakes are centralized repositories for storing and analyzing telemetry data, enabling organizations to derive valuable insights, facilitate long-term analysis, and enhance observability within IT environments.
Chapter 33
Unpacking the Power of Parquet File Format
Parquet file format efficiently stores and processes large-scale data sets, facilitating faster query performance, and enabling cost-effective data storage and analysis within IT infrastructures.
Chapter 34
What is Syslog?
Syslog is a standard protocol used for forwarding log messages within IT systems, crucial for centralized log management, troubleshooting, and security monitoring across diverse networked devices and applications.
Chapter 35
Log Management and Observability in Microservices: Navigating the Challenges
Explore log management and observability in microservices architectures, their importance in facilitating troubleshooting, performance monitoring, and security analysis within distributed and dynamic IT environments.
Chapter 36
Mastering Log Management for Containers- A Step-by-Step Guide
Log management for containers enables effective monitoring, troubleshooting, and security analysis in containerized environments, crucial for maintaining operational visibility and ensuring robustness within modern IT infrastructures.
Chapter 37
Understand Kubernetes Logging
Kubernetes logging is important in tracking containerized application behavior, troubleshooting issues, and ensuring operational visibility within Kubernetes clusters, essential for managing complex cloud-native environments effectively.
Chapter 38
Understanding Platform Engineering: Importance, Current State, and Role of Observability and Telemetry
Platform engineering is a discipline focused on designing, building, and maintaining the infrastructure and tools necessary to support software development and deployment, crucial for enabling scalability, reliability, and efficiency within IT.
Chapter 39
What is an Observability Engineer
An observability engineer is a professional responsible for designing, implementing, and managing systems and processes to ensure comprehensive observability within IT infrastructures, crucial for enhancing operational efficiency and maintaining system reliability.
Chapter 40
What are Grok Patterns
Grok patterns are reusable, pre-defined templates used to parse and structure unstructured log data into a more readable and searchable format. Commonly employed in log management and observability tools, they simplify the extraction of valuable information from logs by matching specific patterns within text data. Grok patterns are essential for efficiently analyzing logs, enabling faster identification of issues and enhancing the effectiveness of monitoring and observability efforts in IT systems.
Chapter 41
Understanding Log Levels: Types, Usage, and Examples
Log levels are categories used to classify the severity of log messages, helping developers and system administrators understand the importance of different events within an application. The main log levels include DEBUG, INFO, WARN, ERROR, and FATAL, each serving a specific purpose in monitoring and troubleshooting applications. Proper usage of log levels ensures that critical issues are easily identifiable, while less severe messages provide context for debugging and analysis, leading to more effective observability and system management.
Chapter 42
Data Normalization - Importance in Observability and Techniques
Data normalization is a crucial process in observability that involves standardizing diverse data formats into a common structure. This ensures consistency and accuracy across logs, metrics, and traces, making it easier to analyze and correlate information from different sources. Effective data normalization enhances system monitoring, troubleshooting, and security, allowing teams to detect and respond to issues more efficiently. Techniques include parsing, enrichment, and categorization, which help in converting raw data into actionable insights for better observability.
Chapter 43
A Guide to Azure Event Hubs
Azure Event Hubs is a fully managed, real-time data ingestion service designed to handle large-scale data streams. It serves as a crucial component in observability by enabling the ingestion and processing of massive amounts of telemetry data from various sources. Event Hubs supports real-time analytics and event-driven architectures, making it ideal for applications requiring high-throughput data streaming. This guide covers its key features, integration options, and best practices for using Azure Event Hubs in observability pipelines.
Chapter 44
A Guide to AWS CloudWatch
AWS CloudWatch is a monitoring and observability service designed to collect and track metrics, log files, and set alarms for your AWS resources and applications. It plays a critical role in ensuring operational efficiency by providing insights into performance and enabling proactive troubleshooting. This guide explores CloudWatch’s core features, including metrics collection, log management, and event-driven actions, and offers best practices for integrating CloudWatch into your observability stack for comprehensive monitoring of AWS environments.
Chapter 45
Understanding Regular Expressions (Regex)
Regular expressions (Regex) are sequences of characters that form search patterns, primarily used for text processing tasks like searching, matching, and replacing strings. They are crucial in observability for parsing logs, filtering data, and identifying patterns across large datasets. This guide explains the syntax, common patterns, and use cases for Regex, highlighting its role in improving efficiency and accuracy in data analysis.
Chapter 46
Logstash: The Backbone of Data Processing in the Elastic Stack
Logstash is a powerful data processing tool in the Elastic Stack, used to collect, transform, and load data into Elasticsearch. This guide covers Logstash's architecture, how it ingests data from various sources, and the ways it transforms data using filters like Grok. It also highlights Logstash's role in the observability pipeline, ensuring that data is structured and enriched before indexing, which enhances searchability and analytics.