Observability 101: Leveraging AI in Modern SIEM Architecture for Proactive Security
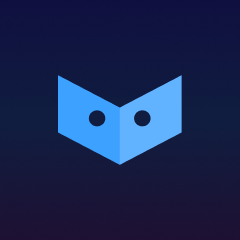
Introduction
In the dynamic and often unpredictable world of cybersecurity, the integration of Artificial Intelligence (AI) and Machine Learning (ML) into Security Information and Event Management (SIEM) systems represents a groundbreaking shift. This blend of technology is steering enterprises from a traditionally reactive security stance to a more proactive and predictive approach. Here, we explore the multifaceted role of AI and ML in enhancing SIEM, their use cases, challenges, and benefits, and discuss the critical role of AI-powered observability pipelines like Observo.ai in optimizing security data.
The Role of AI and ML in SIEM
Transforming Threat Detection and Response
AI and ML algorithms are adept at sifting through massive volumes of data to identify patterns that would be nearly impossible for human analysts to detect in real-time. In SIEM, these technologies can process and analyze vast quantities of security event logs from network devices, endpoints, and cloud systems, identifying potential threats with a level of speed and accuracy far beyond human capabilities.
Proactive Security Posture
AI-driven SIEM systems don't just react to known threats; they learn from data to predict and prevent future attacks. By analyzing historical data, these systems can identify the likelihood of future threats and vulnerabilities, allowing organizations to proactively strengthen their defenses.
Use Cases of AI and ML in SIEM
Anomaly Detection
AI algorithms can identify deviations from normal behavior patterns, such as unusual login attempts or strange data transfers, which could indicate a security breach.
Predictive Threat Intelligence
By analyzing past security incidents and current trends, ML models can predict potential attack vectors and advise on preventive measures.
Automated Incident Response
AI can automate responses to common threats, such as isolating infected devices or blocking suspicious IP addresses, reducing response times and alleviating the burden on security teams.
Challenges in Integrating AI and ML with SIEM
Data Quality and Volume
The effectiveness of AI and ML in SIEM is heavily dependent on the quality and quantity of data. Poor quality or insufficient data can lead to inaccurate predictions and missed threats.
Complexity and Resource Requirements
Developing, training, and maintaining AI and ML models require significant computational resources and specialized expertise, which can be a barrier for some organizations.
Evolving Threat Landscape
The constantly changing nature of cyber threats means that AI and ML models need continuous updates and retraining to remain effective.
Benefits of AI and ML in SIEM
Enhanced Detection and Response Capabilities
AI and ML enhance the ability of SIEM systems to detect complex threats faster and more accurately, thereby reducing the risk of significant damage.
Reduced False Positives
AI algorithms can more accurately distinguish between normal activities and genuine threats, reducing the number of false positives that security teams need to investigate.
Scalability and Efficiency
AI and ML enable SIEM systems to scale with the growing volume of data and security events, while also improving the efficiency of security operations.
The Critical Role of AI-Powered Observability Pipelines
Importance of Optimized and Signal-Rich Data
In this new architecture, AI-powered observability pipelines like Observo.ai play a crucial role in ensuring that the data fed into SIEM systems is highly optimized and signal-rich. These pipelines preprocess and enrich security data, ensuring that the AI and ML algorithms in SIEM systems are working with the most relevant and accurate information.
Enhancing Data Management and Analysis
Observo.ai can intelligently filter out the noise and irrelevant data, streamline data management, and enhance real-time analysis capabilities. This refined data input is crucial for the AI in SIEM systems to perform effectively.
Facilitating Proactive Security Measures
With better data quality and analysis, organizations can shift from a reactive security posture to a proactive one. This shift is essential in today’s fast-paced digital environment, where the cost of a security breach can be catastrophic.
Conclusion
The integration of AI and ML into SIEM systems marks a significant evolution in cybersecurity strategies. This combination offers enhanced detection capabilities, predictive insights, and automated responses, leading to a more robust and proactive security posture. However, the effectiveness of this integration largely depends on the quality of data, highlighting the importance of AI-powered observability pipelines like Observo.ai. These pipelines are instrumental in optimizing the flow of security data, ensuring that AI and ML algorithms in SIEM have the best possible foundation to protect against an ever-evolving array of cyber threats. As we move forward, the synergy between AI, ML, and SIEM will undoubtedly become a cornerstone in the quest for advanced, proactive cybersecurity solutions.