Data First, Tools Second: Rethinking Modern SOC Architecture
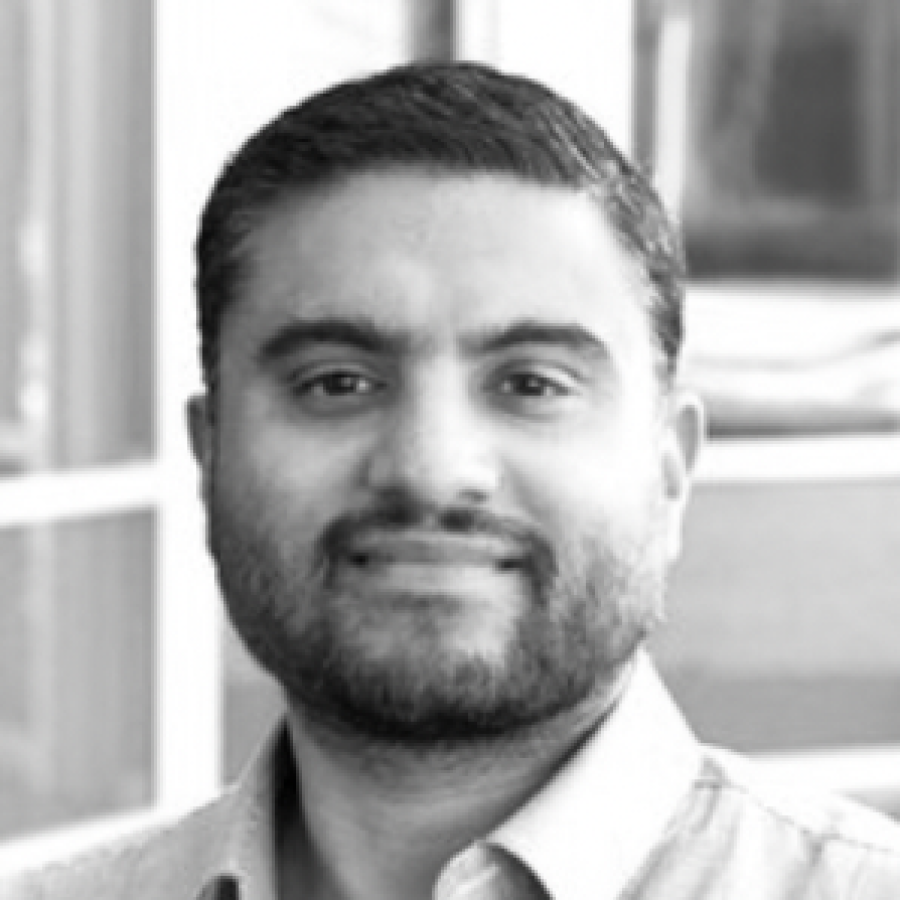
The pursuit of the perfect Security Operations Center (SOC) has become something of a holy grail for security leaders. For years, the industry pushed vendor consolidation as the answer – promising streamlined operations, simplified management, and enhanced visibility through unified platforms. But as the renowned security analyst Francis Odum recently highlighted in his analysis, this approach has delivered more headaches than solutions for many organizations.
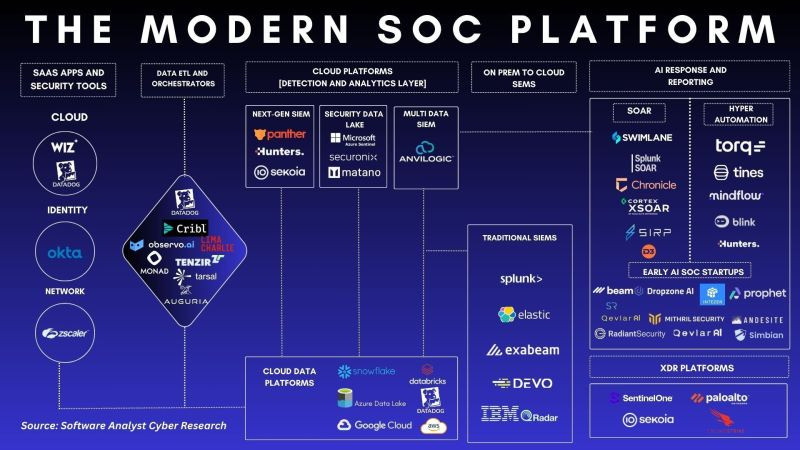
The Broken Promise of Vendor Consolidation
For years, the industry narrative suggested that consolidating security tools under a single vendor would solve our most pressing challenges. Yet today's security leaders find themselves facing an insulting reality: vendor lock-in, spiraling costs, and diminishing returns on their security investments.
The problem isn't with consolidation itself but with where we've been focusing our consolidation efforts. We've been trying to consolidate at the analysis layer – SIEMs, XDRs, and analytics platforms – when we should have been starting at the foundation: the data layer.
Start at the Source: Modernizing Data Ingest
The modern SOC begins with intelligent data management. Before a single alert reaches an analyst, before any dashboard is populated, the data must be properly collected, processed, and distributed. This is where AI-powered data pipelines are revolutionizing security operations.
Why is this the critical starting point? Because the volume of security telemetry has reached unmanageable levels, growing at an astonishing 35% annually for most organizations. By 2025, many enterprises will be processing tens of terabytes or even petabytes of security data daily – an impossible challenge without intelligent automation at the ingestion level.
AI Data Pipelines: The Foundation of the Modern SOC
An AI-powered data pipeline serves as the nervous system of the modern SOC, providing three critical capabilities:
- Intelligent Data Reduction: Machine learning models can identify redundant, low-value data and summarize normal patterns, reducing overall volume by 80% or more without losing critical security signals. This isn't simple filtering – it's sophisticated pattern recognition that preserves essential context while eliminating noise.
- Smart Data Routing: Rather than forcing all data through a single platform, AI pipelines can intelligently route different data types to the appropriate tools. High-priority security events go to premium SIEM platforms, while routine audit logs might be directed to lower-cost storage solutions.
- Real-time Enrichment and Prioritization: By applying sentiment analysis and anomaly detection at the pipeline level, security events can be enriched with critical context and prioritized before they ever reach analysts, reducing the mean time to resolution by up to 40%.
Breaking Free from Vendor Lock-in
One of the most significant advantages of implementing an AI data pipeline is the liberation from vendor lock-in. As Odum points out, many organizations find themselves captive to their SIEM providers, facing astronomical costs during contract renewals with few viable alternatives. By taking control of the data layer, security teams gain the flexibility to evaluate and integrate new tools without vendor-imposed constraints.
By decoupling data ingestion from analysis, security teams regain control of their technology stack. Want to test a new SIEM? No problem – simply route a copy of your optimized data to the new platform. Need specialized analytics for cloud security? Direct relevant telemetry to purpose-built tools without duplicating data collection efforts.
This flexibility allows security leaders to build best-of-breed solutions tailored to their unique needs, rather than accepting the limitations of a single vendor's vision.
The Economics of Intelligent Data Management
The financial impact of AI-powered data pipelines cannot be overstated. Organizations implementing these solutions typically report:
- 50-70% reduction in total SIEM and storage costs
- 30-40% improvement in analyst efficiency
- 40% faster incident resolution times
These aren't marginal improvements – they're transformative changes that free up budget for strategic security initiatives while improving overall security posture.
Beyond Traditional ML: Understanding Agentic AI in Security Operations
With virtually every security vendor now claiming "agentic AI capabilities," it's crucial for security leaders to understand what this term actually means beyond the marketing hype.
It's important to differentiate between agentic AI and AI agents. Essentially, agentic AI is the framework; AI agents are the building blocks within the framework. Agentic AI is the broader concept of solving issues with limited supervision, whereas an AI agent is a specific component within that system designed to handle tasks and processes with a degree of autonomy. This model is changing how humans interact with AI.
In security operations, this distinction matters tremendously. Agentic AI isn’t just automation with natural language processing—it represents an entirely new paradigm in security operations. True agentic systems in security operations demonstrate:
- Goal-oriented reasoning: The ability to understand the security objective (e.g., "identify potential data exfiltration") and independently determine the necessary steps to investigate
- Multi-source intelligence synthesis: Combining signals across disparate data sources to form cohesive security insights without predefined correlation rules
- Adaptive response selection: Choosing appropriate actions based on context rather than rigid playbooks
- Continuous learning: Improving performance through operational feedback without requiring constant retraining
For CISOs evaluating security tools with AI capabilities, the distinction represents more than semantic preference. Investing in platforms that merely repackage traditional automation as "agentic AI" can lead to significant setbacks—both in terms of wasted resources and missed security opportunities. The most effective modern SOCs are building their strategies around genuine agentic capabilities that augment human expertise rather than making grandiose claims about replacing it.
When properly implemented at the data pipeline level, genuine agentic AI can transform security operations by handling routine analysis at machine speed while elevating human analysts to focus on complex investigation and strategy. This is where the true ROI of modern security operations emerges.
The Path Forward: Modernizing Your SOC
For security leaders looking to modernize their operations, the message is clear: start with your data foundation. Before investing in new analytics platforms or considering vendor consolidation, implement an intelligent data pipeline that gives you control over your security telemetry.
This approach provides immediate cost savings while creating the flexibility to evolve your security stack over time. Instead of being forced into disruptive "rip and replace" scenarios, SOC teams can transition to more efficient, specialized tools while maintaining operational continuity.
The modern SOC isn’t defined by a single vendor—it’s defined by intelligent data orchestration. By implementing AI-powered data pipelines at the foundation of your security architecture, you gain the freedom to choose the best tools for each purpose while optimizing costs and efficiency.
Conclusion
The next generation of security operations centers will be defined not by vendor consolidation but by intelligent data orchestration. By implementing AI-powered data pipelines at the foundation of your security architecture, you gain the freedom to choose the best tools for each purpose while controlling costs and improving efficiency.
With security telemetry volumes growing by 35% annually and threat actors continuously adapting their techniques, this data-first approach isn't just financially prudent – it's a strategic imperative. Tomorrow's successful security teams won't be defined by which vendor they chose, but by how effectively they harnessed their data to drive meaningful security outcomes.